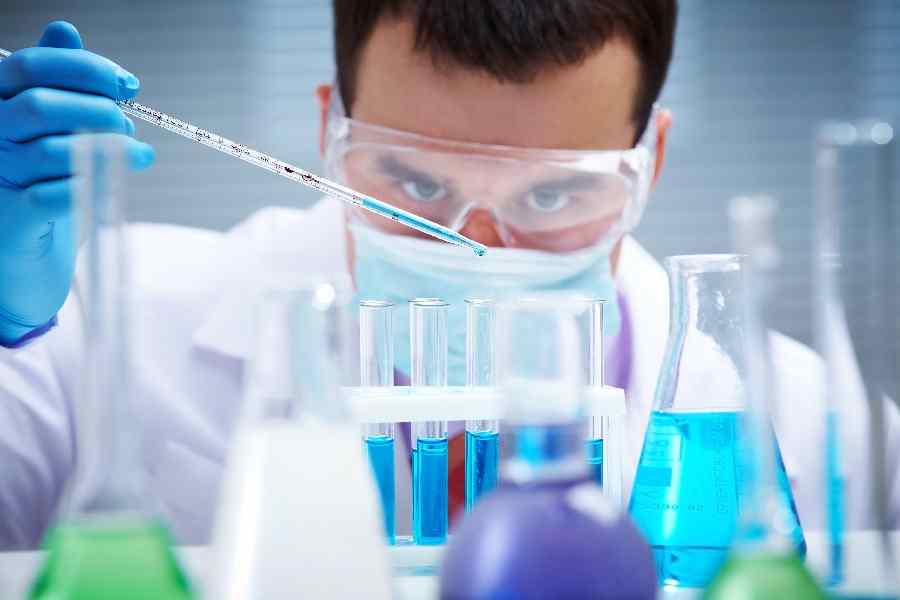
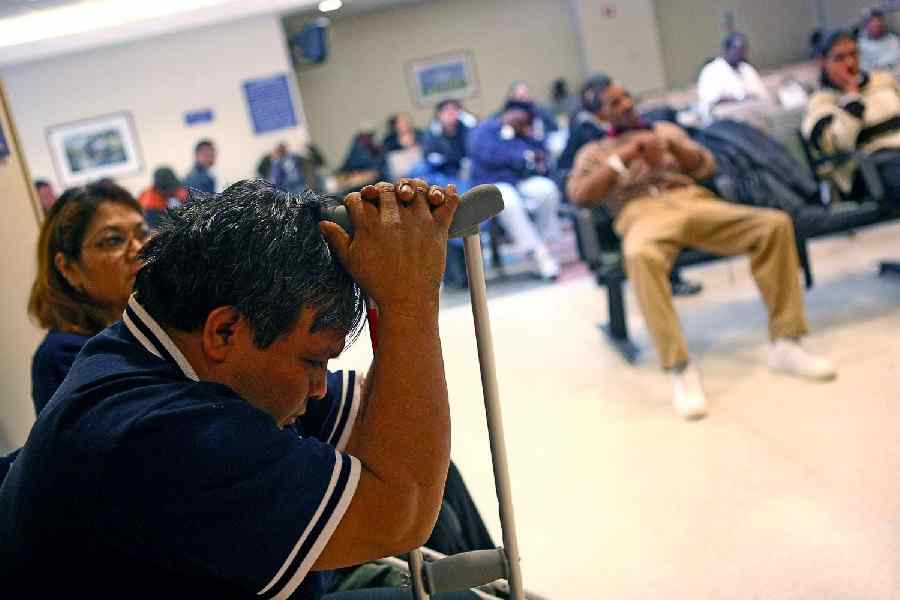
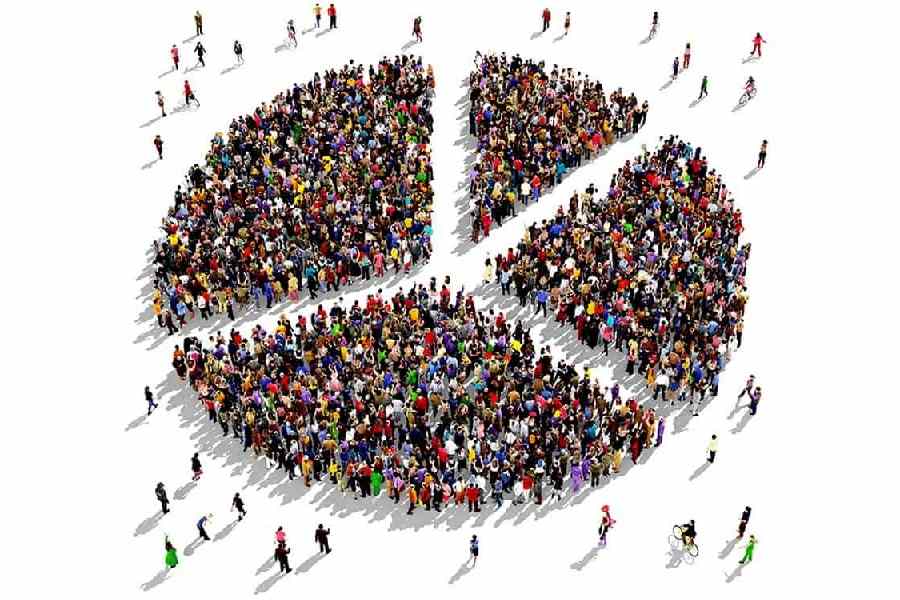
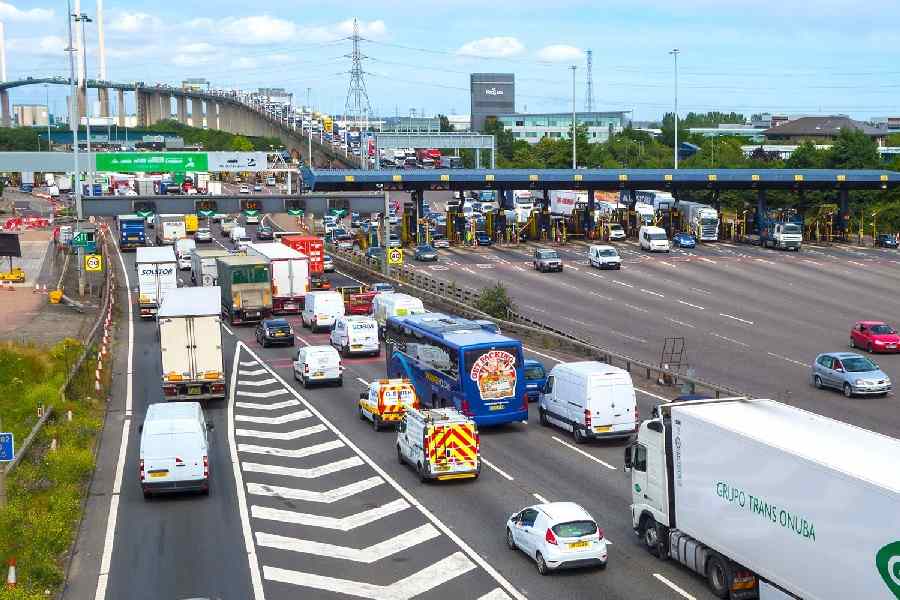
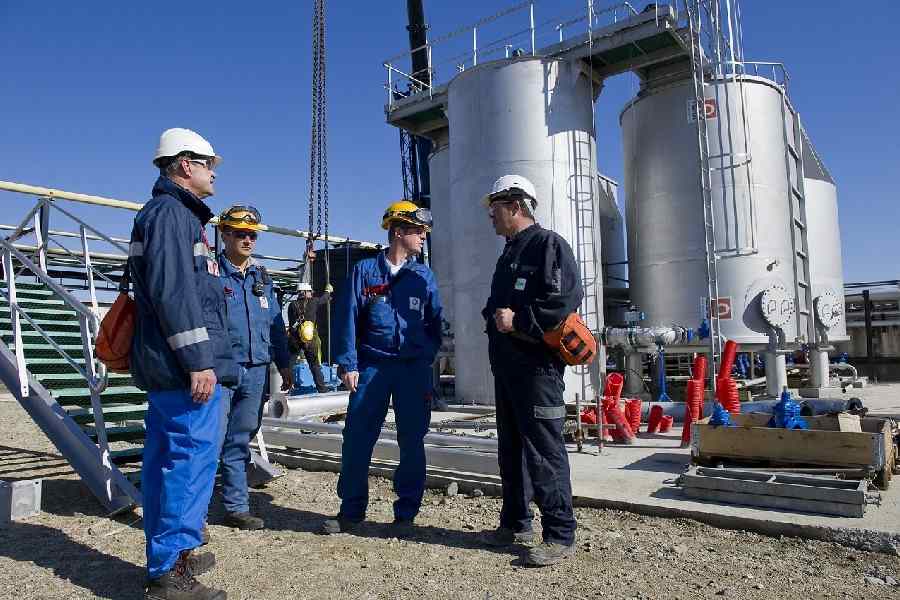
Operations Overhead Cost Reduction
Data Science for Operations Monitoring and Cost Reduction
Read MoreChemical Concentration Prediction
Data Science in the Petrochemical Industry
The Problem
- Quality Control Process: Identification of chemical and compound concentration in a product
- Cost of chemical analysis of individual chemical and compounds varies.
- Measurement of some chemicals is expensive
The Objective
- Reduce cost of chemical analysis.
The Solution (Automated AI and Machine Learning)
- Build hundreds of predictive models.
- Individual and ensembles.
- Optimize models.
The Result
An optimized model that predicts concentration of “expensive” chemicals based on the concentration of “cheap” chemicals. Accuracy ~ 94.2%
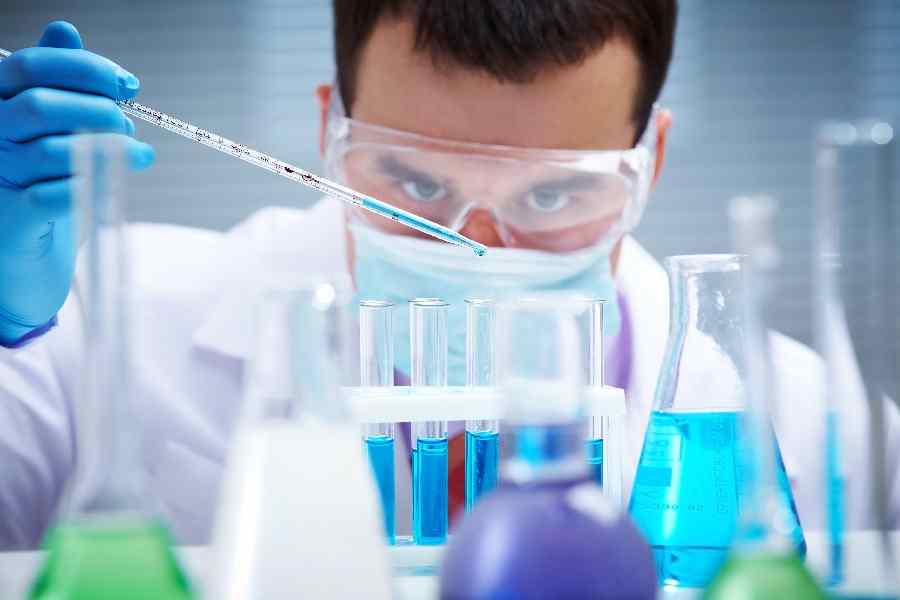
Prediction of ER Patient Waiting Time
Data Science in the Healthcare Industry
The Problem
- Patients registering at ER desk in a hospital get frustrated from waiting times.
- Frequent questioning of counter staff poses hurdles in ER work.
The Objective
- Improve client experience while waiting.
The Solution
- Process historic data of ER visits, patient history and resource utilization.
- Build hundreds of predictive models.
- Individual and ensembles.
- Optimize models.
The Result
An optimized ensemble of models that predicts waiting time for a patient. Accuracy ~ 86%
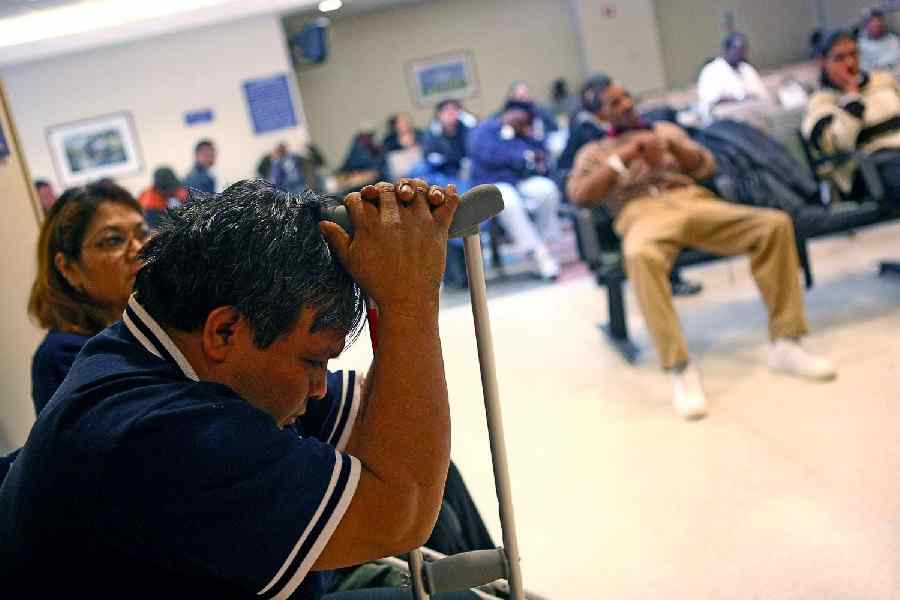
Customer Segmentation (for marketing)
Data Science in Marketing
The Problem
- Company has over 10M customers.
- Company has a large bag of products and services.
- Company wants to conduct targeted advertising campaign to sell a specific product.
The Objective
- Identify top 10,000 potential customers.
- Instruct the telemarketing department to promote a chosen product.
The Solution
- Analyze customer usage history.
- Capitalize on demography data and survey results (provided by client).
- Automated Data Mining.
- Identify association patterns.
- Cluster search.
The Result
Provide list of potential customers. Acceptance Rate ~ 72% (reported by client)
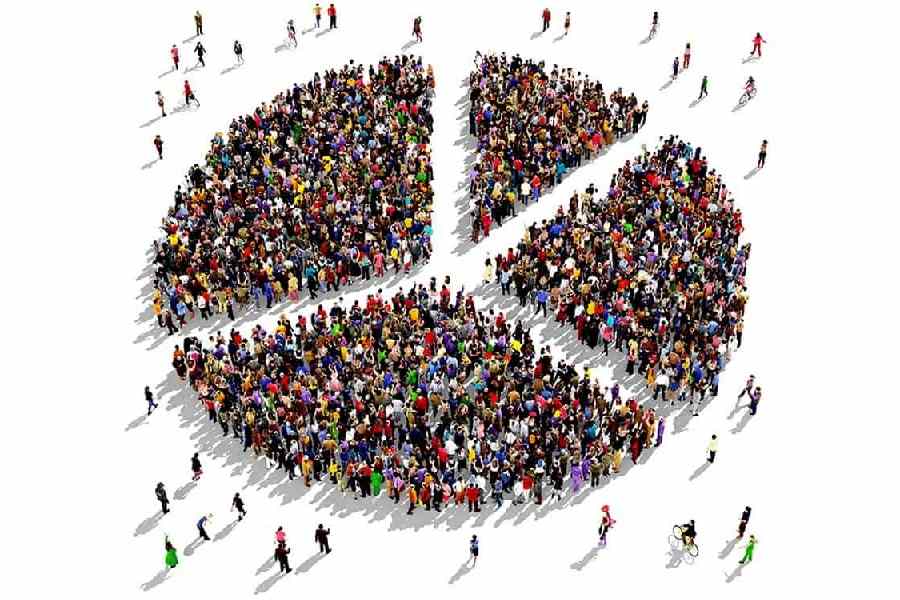
Variable Toll
Data Science for Traffic Control
The Problem
- Narrow traffic corridors often experience congestion during peak hours. Especially on toll booths.
The Objective
- Distribute traffic evenly throughout the entire day by linking toll price with predicted traffic.
- Optimize toll booth operations.
The Solution
- Analyze traffic and demographic data of travelers.
- Build hundreds of predictive models.
- Individual and ensembles.
- Optimize models.
The Result
An optimized prediction model to predicts traffic at narrow corridors of a national highway. Theoretical Accuracy ~ 91.2%
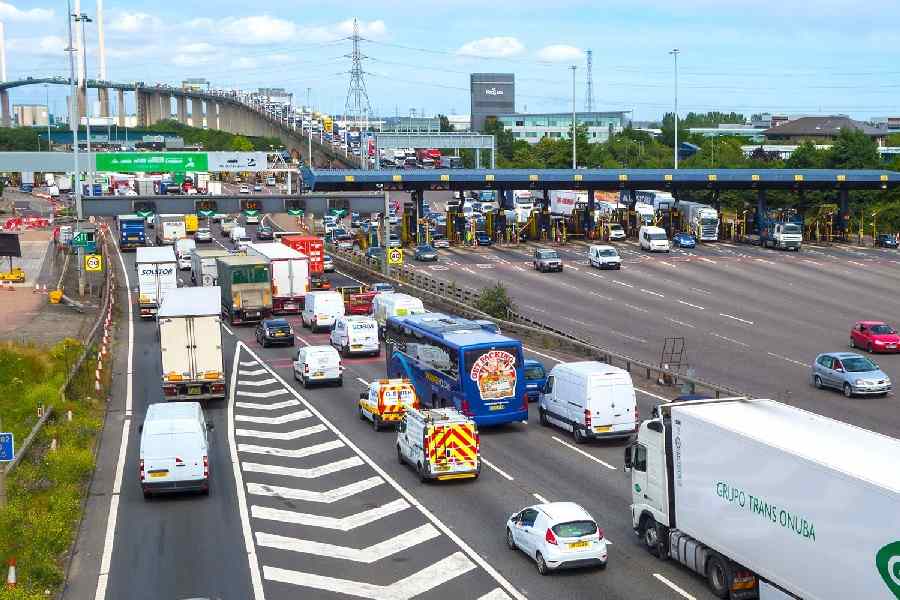
Operations Overhead Cost Reduction
Data Science for Operations Monitoring and Cost Reduction
The Problem
- Client has grid of sensing stations over a vast geographic area.
- As the grid grows, so does the operation, monitoring and maintenance costs.
The Objective
- Reduce grid operation cost by eliminating grid points.
The Solution
Automated AI and ML- Build hundreds of predictive models for each grid point.
- Identify grid points for elimination.
- Replace eliminated grid point readings with predictive model.
The Result
Propose candidate grid points for replacement with prediction models. Cost reduction ~ 37%
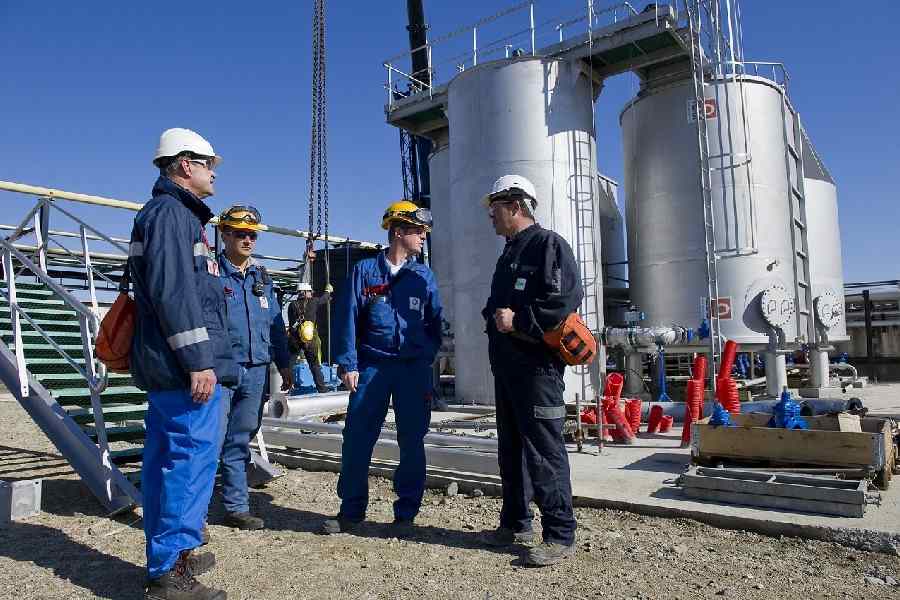